Demand-Supply Optimization
By accurately predicting rental car prices (through these predictive models), insurance companies can anticipate periods of high demand and secure rental cars in advance. This proactive approach ensures that they have an adequate supply of vehicles available when claimants require them. It helps avoid delays in the claims process and enhances customer satisfaction.
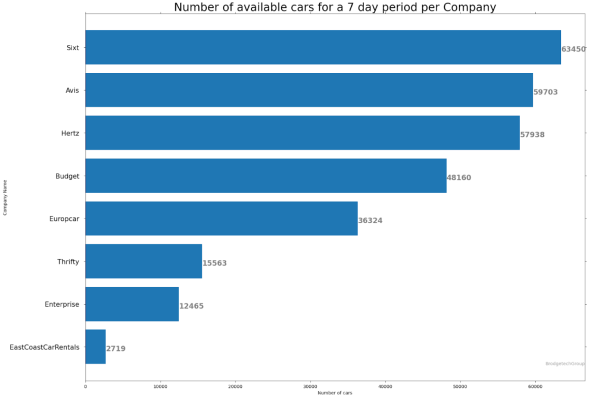
Cost Savings
Predicting rental car prices allows insurance companies to assess the potential costs associated with car rentals. By understanding the expected prices for rental cars, they can better estimate the financial impact of claims and allocate appropriate reserves. This enhances their risk management capabilities and helps them plan and budget accordingly.
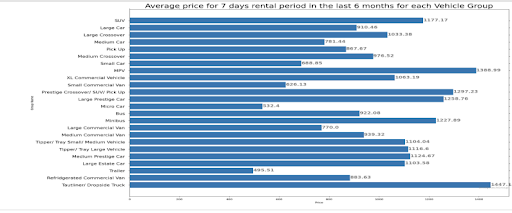
Comparison Shopping
With the ability to predict car rental prices, consumers can compare prices across various rental companies and make informed choices. They can identify periods when prices are expected to be lower and select the most affordable option without compromising on their requirements. Predictions provide valuable insights into the market, enabling consumers to find the best.
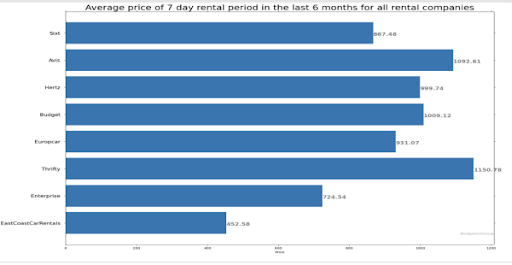
Performance
Good Communication & Customer Support
With rental car price forecasts, insurance companies can inform claimants about the expected reimbursement amounts based on the forecasted rental car prices, ensuring transparency and managing customer expectations.
Competitive advantage
Having the ability to predict rental car prices gives insurance companies a unique competitive advantage. It sets them apart from competitors by enabling them to offer more accurate and fair claim settlements.
Fraud Detection
Insurance fraud is a significant concern for insurance companies. A price prediction algorithm can help identify suspicious rental patterns or abnormal pricing behaviors that may indicate fraudulent activities.
Anomaly Detection
We determined that some values are abnormal based on Time Series Models for Anomaly detection. Below, we can see that the spikes from the original series have been highlighted in orange, raising this way some anomalies each time the number of cars increased significantly compared to the previous values.
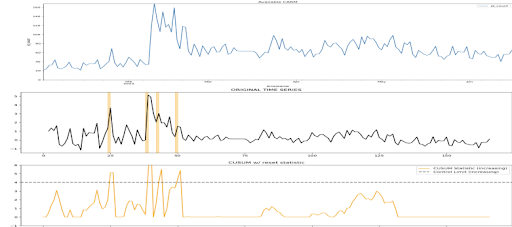